Everything Data: The Evolution of Data-Driven Decision Making
By Jay Yeo, Data Analyst, ORI
Working with data is never done. Utilizing data to drive decisions is an ongoing process that requires a comprehensive approach to data—always. We have found that supporting organizations as they grow and progress toward data-driven decision making requires a wide range of skills to guide clients successfully through the iterative processes of data collection, benchmarking, and analysis that are necessary to become truly data-driven.
Data Collection
In order to become data-driven, first, organizations must have data relating to customer and prospect wants and needs. Collecting data can look different depending on the organization and context and may involve a combination of strategies ranging from surveys to customer forms and more. We find that many of our clients rely on four key strategies for collecting data:
- Customer and member profiles: Basic information that customers or members must submit in order to become customers or members.
- Surveys: Periodic questionnaires distributed to either all or a segment of customers or members designed to attain information on key data points or issues.
- Social media and online forums: Organic interactions of customers or members with the organization or with one another about organization-related themes.
- Call centers: Notes fields and recordings of calls between customers or members and the organization.
Data collection, it should be noted, is not a one-time exercise. Rather, it is an iterative process that evolves as market and customer trends shift and customer demographics change—which, in turn, require regular check-ins and updates. Organizations do not stay the same year over year, so why would their customer and member data remain unchanged?
Data Benchmarking
Once data is being collected regularly but before it is being utilized to drive decisions, organizations should check the health of their data to ensure that any insights derived from it will be sound. Each data benchmarking exercise differs depending on the context, but broadly, the process involves checking key data fields for a minimum of completeness, validity, and timeliness. This process may reveal areas of weakness that require either gap filling or additional data collection before the data will be usable for analysis.
Our clients often find that this step of the process intersects with data collection as well as data analysis. A key reason for this overlap is the need to clearly define data analysis goals. As organizations shift from focusing on the initial collection of data to defining the ways that data can inform their decision-making process by determining the key fields to be used for benchmarking, they often uncover gaps between what they have and where they want to be. For this reason, data benchmarking is often a transitional step for organizations moving from solely collecting data to also analyzing data by applying careful thought and planning.
Data Analysis
Once an organization has a healthy repository of data and clearly defined goals, it can begin to utilize its data for analysis to gain insights that can be transformed into action. Data analysis itself is comprised of several key layers that build upon one another to eventually enable an organization to utilize its data to its fullest potential. While many similar visuals exist, we have found Presbyterian Healthcare Services’ illustration of these five layers of analytics maturity to be the most reflective of the client experience:
- Descriptive: What happened?
- Diagnostic: How many, how often, and where?
- Predictive: What will happen next?
- Prescriptive: What actions are needed?
- Optimization: What is the best I can do?
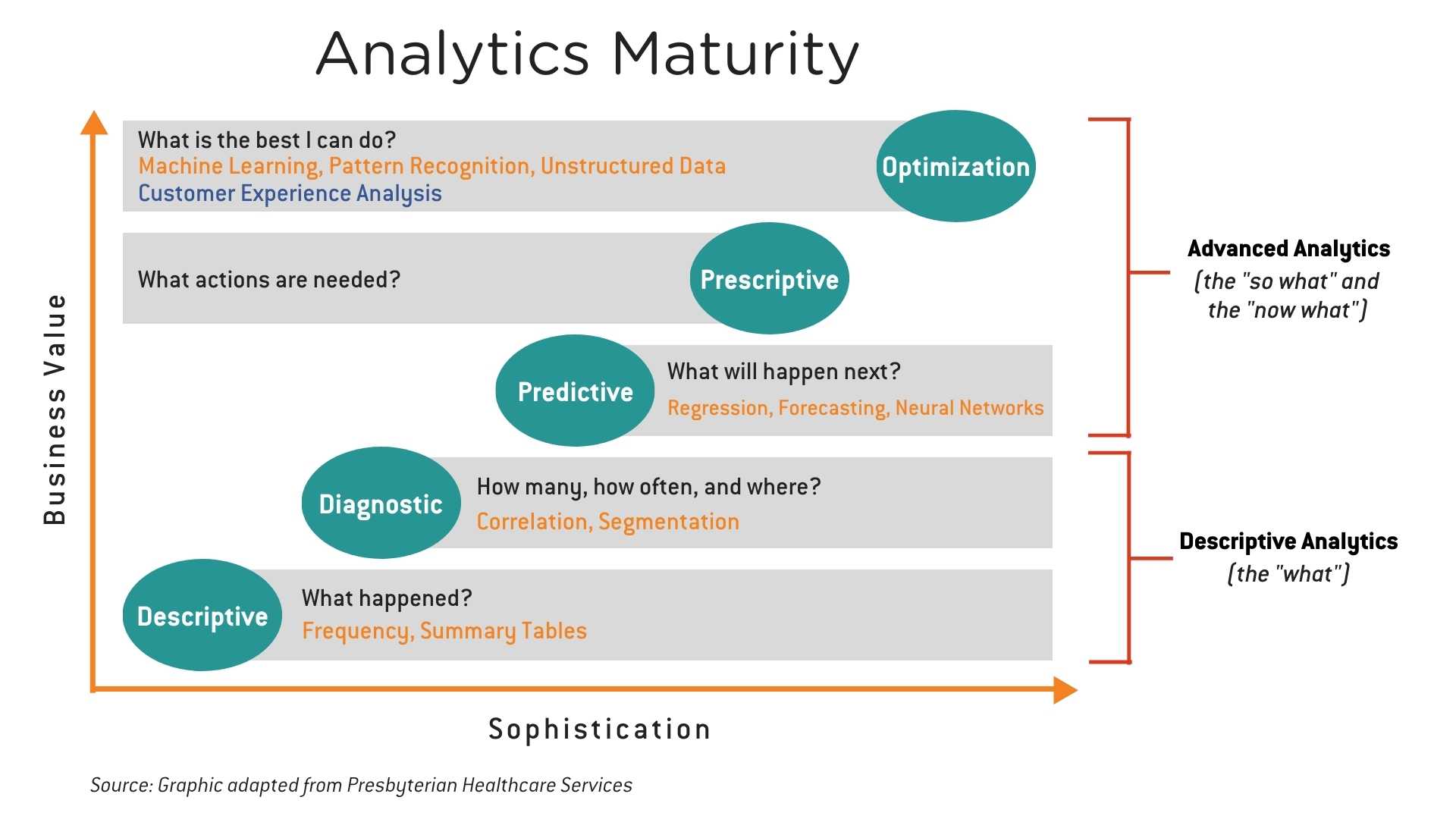
Like data collection and benchmarking, adding layers to data analysis is also an iterative, ongoing process that intersects with these exercises. If an organization has fewer healthy data pools, a simpler analysis may be the only feasible option at the beginning stages. As more healthy data pools are added through both data collection and data benchmarking, the organization may begin to deepen its analysis through these five layers to ultimately arrive at optimization.
Achieving the final stage of data analysis—optimization—requires that an organization not only have significant data resources but that any walls or siloes separating the data be destroyed. Often times, organizations have rich repositories of data but, because it is siloed, they are limited in their ability to use the full breadth of available data. By achieving this last and final layer, organizations are able to utilize all of their data—including often neglected but insight-rich unstructured data—in combination with one another to provide both far-reaching and detailed insights to drive action.